How Google DeepMind is Using GenAI to Change Weather Forecasting Forever
Plus, Twilio founder Jeff Lawson and Supercell founder Ilkka Paananen offer their hard-won wisdom to startup founders
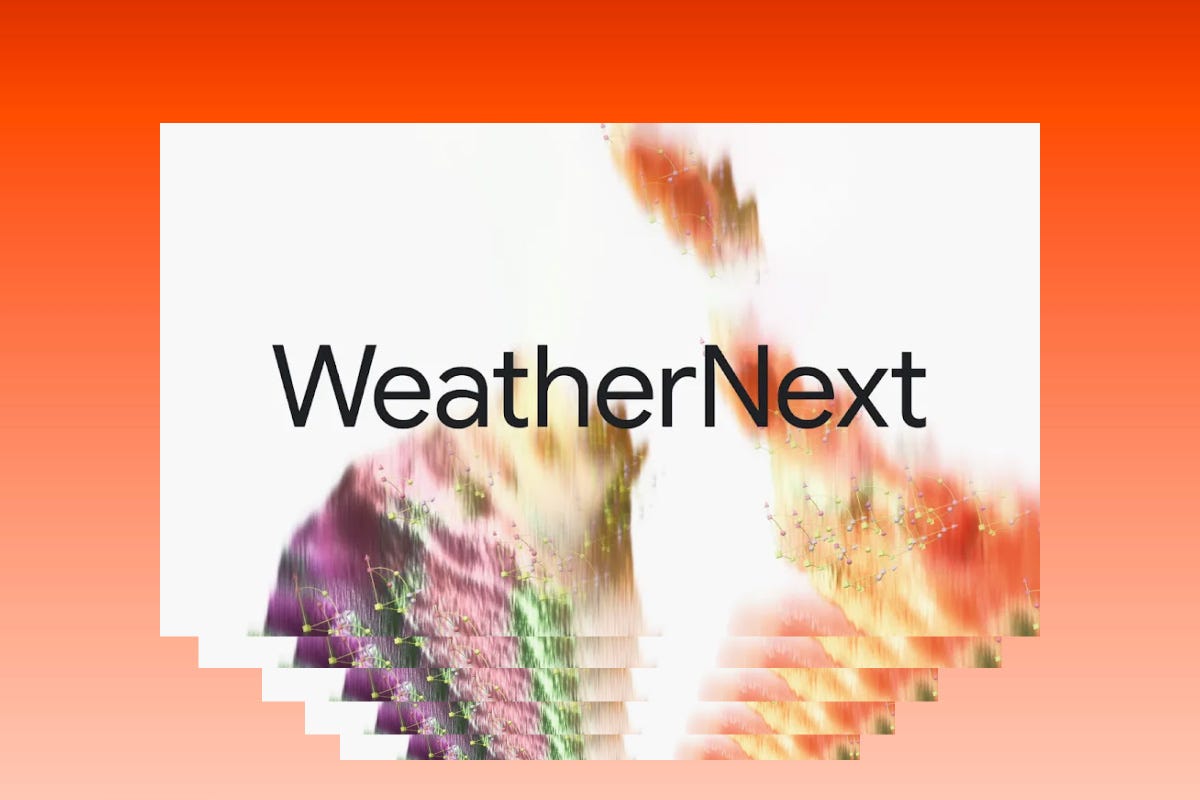
So much of the public conversation about AI revolves around its future potential.
You’ve seen the debates: Some fret about possible chaos in the job market. Others foresee a coming age of abundance. Because the tech is evolving so quickly, it’s tempting to focus only on what it could mean.
But there are already astonishing new applications emerging from current AI models.
Take for instance WeatherNext Gen, Google DeepMind’s latest AI-powered weather prediction model that’s capable of producing astonishingly accurate forecasts up to 15 days out. It offers a leap forward in weather prediction that, according to the DeepMind team’s original GenCast paper published in Nature in December, is faster, leaner, and more accurate than the world’s previous best-in-class models. This sort of predictive technology can improve the reliability of renewable energy, de-risk supply chains, and even save lives.
None of this required an artificial superintelligence. Instead, WeatherNext Gen is a diffusion model. It leverages some of the same tech behind the GenAI image, video, and music apps you’re already familiar with.
It’s not obvious that GenAI tech could be useful for predicting the weather. But Matthew Willson, a Staff Research Engineer at Google DeepMind and one of the co-authors of the DeepMind paper in Nature, tells speedrun that there are surprising similarities between video generation and weather prediction.
“In a crude analogy, we can think of a weather reanalysis dataset like ERA5 as a gigantic, many-channel, 4-dimensional video of the atmosphere,” Wilson says. “Thus we were able to adapt some diffusion methods used for conditional video generation, to generating weather forecasts.”
There are differences, of course. To name one, weather forecast models require you to generate spherical geometry that wraps around the Earth’s surface. And, Wilson says, individual frames of global weather data are massive files—bigger than any frame from a 4K video.
“Weather is harder in some ways and easier in others than generating video,” Wilson says. “But the tasks are similar enough that some research methods can be shared.”
From Nowcasting to Forecasting
The Google DeepMind team has spent years experimenting with using deep learning tech to improve weather forecasting. In 2021 they introduced a model trained on radar data that improved short-term precipitation forecasts with a 5-90 minute lead time—a field of weather prediction sometimes called “nowcasting.”
The team was encouraged by the results they got in these short-term prediction systems, but Google’s Wilson says there was “significant skepticism” at the time about the limits of machine learning techniques when it came to beating out more traditional weather forecasting models.
But other teams, including those at Google Research, began drawing attention to new benchmarks for forecasting models. “We decided our next challenge would be to tackle this bigger global medium-range weather forecasting task,” Wilson says. This work resulted in a paper in Science from the DeepMind team that introduced a new weather model called WeatherNext Graph that’s deterministic (meaning it generates just one forecast).
The DeepMind team then set out to develop a probabilistic model—one that could show many possible futures instead of one “likely” one. “For a long time,” Wilson says, “we have believed that machine learning solutions for weather should model uncertainty in a principled way.” Using a diffusion AI model offered a promising route forward, and the team ultimately settled on a model that generates 50 possible weather forecasts at once.
This diffusion-powered approach has blown existing forecasting models out of the water. The DeepMind team says that WeatherNext Gen was more accurate than the current gold standard operational system “on 97.2% of these targets, and on 99.8% at lead times greater than 36 hours.”
Extreme Weather and the Many Possible Futures of Forecasting
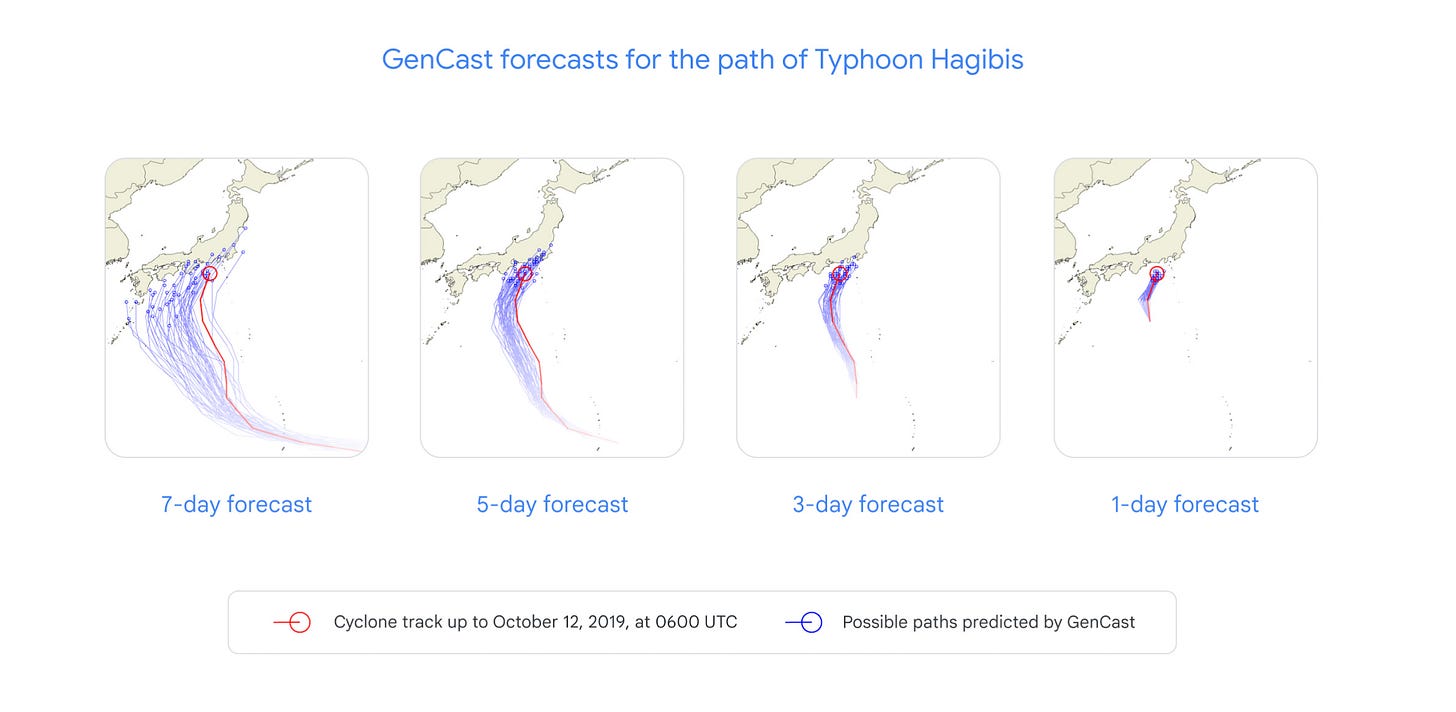
There are obvious benefits of better long and midrange weather forecasting, but the holy grail would be if probabalistic, AI-powered models also allows us to better prepare for rare and extreme weather events. And there are already signs that models like WeatherNext Gen are better at prediciting extreme temperatures, extreme winds, and trajectories of tropical cyclones and hurricanes.
Alvaro Sanchez-Gonzalez, a Staff Research Engineer at Google DeepMind, says WeatherNext Gen is faster and requires fewer computational resources to generate predictions. “This means that with a bit more resources, it could be used to generate, not just 50 alternative future predictions, but many more, potentially hundreds or even a thousand,” Sanchez-Gonzalez says. “The hope is that this will make it easier to predict extreme phenomena that occur with lower probabilistics more in advance than traditional approaches.”
Sidebar: Can GenAI Techniques Be More Efficient Than Traditional Computing?
Sanchez-Gonzalez’s statement that WeatherNext Gen requires less compute than traditional weather forecasting might strike some as surprising, given the reputation GenAI tech has for being very compute demanding.
We asked if he could elaborate, and found the math fascinating:
“We train and run WeatherNext Gen on Tensor Processing Units (TPU). TPUs are specialized hardware to train all kinds of AI, much more efficiently than using a regular PC, but with a similar size. Similarly to how the graphics card of a regular computer (also known as GPU) is specialized in rendering images, TPUs are specialized in making matrix products very very fast. We train WeatherNext Genusing 32 of these TPUs for about 5 days, and once trained, a single TPU can produce a 15 day forecast in just 8 minutes, and 50 of these can be used to produce an ensemble of 50 forecasts in parallel. This contrasts with the traditional approaches which take about 2 hours to produce a similar set of 50 forecasts using a supercomputer with tens of thousands of processors.”
—Alvaro Sanchez-Gonzalez, Staff Research Engineer at Google DeepMind
The DeepMind team believes that there are big gains still be discovered for generative AI powered forecasting systems like this one, in part because they’ve achieved their current results with relatively limited data.
“The catalogue of available weather data is enormous and in this work has only tapped into one kind of weather data: which is the global analysis data provided by ECMWF, without which this model would not have been possible,” says Sanchez-Gonzalez. “However, there are many more possible data sources that we are seeing other AI weather models start to use, such as direct satellite images, sensors, radars, etc.”
Of course, when building tools to predict the future, there will always be limits. “At some point we will hit the barrier of predictability, and it will be hard to move past it,” Sanchez-Gonzalez says. “However, the good news is that we have not yet seen evidence of having hit that barrier yet!”
The DeepMind team has released the code and weights for WeatherNext Gen on its website, and says they hope to start seeing third party integrations available to consumers this year.
Wisdom for founders from Twilio’s Jeff Lawson and Supercell’s Ilkka Paananen
Twilio founder (and owner of The Onion) Jeff Lawson famously took Twilio public with only 12 salespeople on staff. Speaking in person to a group of a16z speedrun founders last week, he told how a painful experience working with Uber taught him the real value of salespeople and led him to dramatically expand his sales org.
“They called us one day and said ‘hey, we just want to let you know, we think we’re gonna cut our Twilio bill in half…’ They were our biggest customer, and we’d just gone public two quarters earlier. So that’s an event you have to disclose to investors right away… Our stock lost 40% of its value in one day. And the thing that I learned from that… is that the salesperson is not for them. It is for you! You need to know that this customer is locked in, loves you, that you’ve got your ear to the ground… so that whatever is going on you know and you’re ahead of it. And we just weren’t.”
—Twilio founder Jeff Lawson
Supercell founder and CEO Ilkka Paananen explained why Supercell embraces a radical bottoms-up approach to game development that empowers individual game teams over leadership.
“Most game companies are super hierarchical, meaning essentially that the CEO or the leadership team decided ultimately what type of games the company would build… It’s basically based on this assumption that somehow the CEO or the leadership team has a crystal ball and really knows what the players or consumers want. And then I had this moment of realization… that despite all of the great processes I had put in place, [and while] we were able to put out some great games, all of those great games came somehow outside of that official process.”
—Ilkka Paananen, Founder and CEO of Supercell
💡 More Big Ideas
🤖 Voldex—an a16z Games fund portfolio company—became the Roblox developer with the largest overall audience after acquiring Brookhaven. Our own Andrew Chen recorded a short interview with Voldex founder and CEO Alex Singer.
🐋 a16z’s own Alex Rampell wrote an op-ed in The Free Press that explained Why DeepSeek Is a Gift to the American People. “China’s AI breakthrough has exposed our policy failures,” wrote Rampell, “And not a day too soon.”
📈 speedrun GM Josh Lu argues that “Good” metrics aren’t as impressive as you think, and even worse they can trick you into believing you’re on the path to PMF. “Great” is the only thing that matters.
📱 Andrew Chen published a viral essay about the phenomenon of easy-come-easy-go users in the age of social media: My product went viral on social media but all I got were these shitty users
💼 There are currently over 400 open jobs listings across our portfolios. Join our talent network for more opportunities. If we see a fit for you, we'll intro you to relevant founders in the portfolio.
That’s it for this week. If you haven’t already subscribed, it’s time to lock in. We’ll see you next Tuesday.